Beyond content moderation - implementing algorithm standards and maintaining free speech
- Jeff Hulett
- Oct 26, 2021
- 7 min read
Updated: May 26, 2022
An approach to balancing free speech protection and hate speech control
(First published, December 2020)

In the book Dare To Speak by Suzanne Nossel, the author makes a thoughtful case for holding technology platform companies (e.g., Google, Facebook, Twitter) accountable for their influence on public discourse. Nossel describes platform company processes, approaches, international human rights agreements, and U.S. law as part of the “content moderation” infrastructure. The concept is to appropriately moderate content in a way that suppresses illegal or “immoral” content, identifies questionable content, and ensures freedom of expression for the remaining content. The author is a free speech advocate and accepts the reality that technology platform companies are the new marketplace of ideas. A marketplace that is subject to the First Amendment but, nonetheless, represents challenges never anticipated by the framers of the U.S. Constitution or Bill of Rights. This article accepts the marketplace of ideas premise but suggests a different approach to holding platform companies accountable. Our suggested approach targets the source of the content promotion itself. That is, the algorithms big tech companies rely upon to promote content.
Algorithms - the secret sauce
Newer technology platform companies' competitive advantage “secret sauce” is found in the algorithms they utilize to drive user engagement. Academic research, including supporting evidence from Rathje, et al (1), demonstrates that emotion-laden response, often with negative sentiment, is at the objective core of the engagement driving algorithms. The algorithms are forms of Artificial Intelligence and Machine Learning that target human neurological functions, especially as it relates to our addictive proclivity. Through the use of unsupervised learning, the machines learn to “hack” human neurological functions to optimize view time. The optimization algorithm presents content, while increasing extremity, to satisfy the addictive effect. As such, for an individual to receive the same dopamine-induced sensation and for the platform to attract or maintain engagement, the individual must receive increasingly extreme content. The same addictive effects are evident in parallel activities, like gambling, smoking, alcohol, and drugs.
In an ironic twist, the parallel addictive activities are all heavily regulated or expressly illegal, especially as it relates to children. Whereas social media remains mostly unregulated and is regularly available to children.
No wonder the technology platform firms have been utilized by political parties and hate groups. Both of which deliver polarizing content to recruit and maintain membership. Both have been known to create social media “echo chambers,” where extreme content is amplified, with operational reinforcement by the algorithms.
Social Media companies know the “like”-tuned, oxytocin-targeted, and dopamine-producing algorithms are optimized to deliver more likes, engagement, and advertising revenue. We present the neurotransmitters oxytocin and dopamine as core algorithm-induced chemical agents, interacting with our brains neurological function to render a “like” response. In particular, see the following “High emotion tag / low language case” as a model for how social media algorithms seek to bypass the left hemisphere and executive function control to drive emotion-based responses. (2)
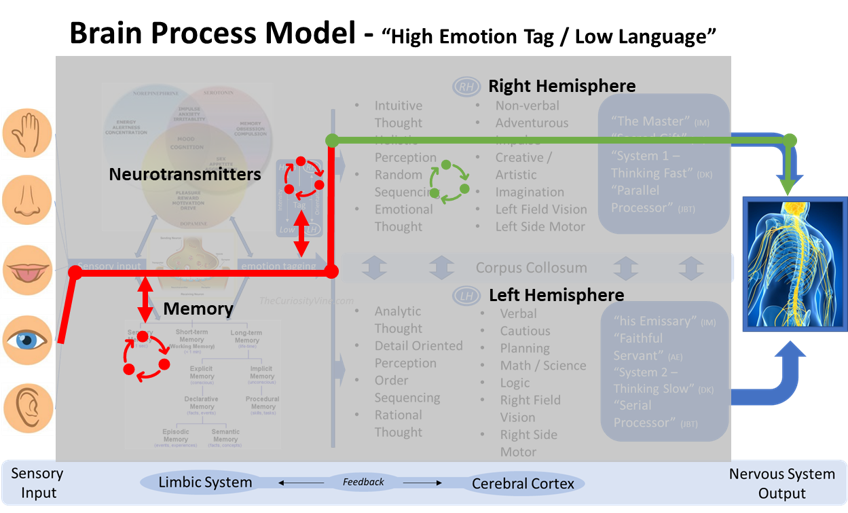
In the end, the better the algorithm is at serving more extreme content, the more advertising revenue is generated. To this point, in 2019, about $300 Billion was spent on internet advertising. This represented doubling of internet advertising spend over the previous 4 years. Therefore, technology platform firm’s have a tremendous incentive to continue algorithm-enabled neurological hacking. (3)
As such, society needs to address the algorithms themselves. (4) The algorithms should be required to be transparent and there should be limits placed on the algorithms ability to increase the extremity of the presented content. The need is to implement algorithm standards as it relates to exploiting the human evolutionary condition that makes us vulnerable to addiction and extremism. In particular, our children need algorithm standards as their developing brains are most vulnerable. This should be completed in the context of “not throwing the baby out with the bath water.” Technology platform firms create economic value, jobs, and a marketplace for useful ideas and communication.
Algorithm Standards Examples
The following are 2 operating examples for algorithm standards. These examples have been successfully implemented in related financial services market contexts.
Speed Bumps Example:

High Frequency Trading (HFT) is considered a comparable “race to the bottom” competitive problem as found in the technology platform industry. (5) The HFT systemic issue is the reinforcing competitive need to increase order execution speed in the high stakes, high-profit financial securities business. As a countermeasure, Brad Katsuyama and the Canadian firm IEX, (6) developed a securities exchange to add systemic “speed bumps.” In effect, speed bumps are a balancing control that places securities traders on the same (slower) speed, creating an even trade execution playing field and reducing the incentive for more extreme speed.
As an analog for the technology platform algorithms, “speed bumps” could be implemented that limit the technology platform’s ability to target and present increasingly extreme content. This is intended as a content moderation approach but is directly tied to algorithm operations. Perhaps, a limit of only one additional optimized view before the algorithm is disengaged from the content optimization process. Or, perhaps the algorithm predictor is changed from engagement to a variable less likely to drive extremism, like related information value. For example, if you are in a hate group, the new “speed bump” predictor would be just as likely to suggest content with arguments against hate speech as for hate speech. If new “speed bump” laws could be passed and the associated rules could be written, new startups or existing firms could develop and commercially implement such speed bump technology.
Outcome-Based Example:

Bias in mortgage lending is considered a comparable information control problem as found in the technology platform industry. Mortgage lending is already regulated by a variety of laws and regulations. In the U.S., this includes Fair Lending, the Home Mortgage Disclosure Act (HMDA), the Equal Credit Opportunity Act (ECOA), and the Fair Credit Reporting Act (FCRA). (7) These laws regulate loan-related bias via an outcome-based approach. That is, HMDA testing is required to ensure certain lending biases against defined protected classes (e.g., race, gender, age, sexual orientation, etc.) are monitored and, if detected, are corrected. For example, if a loan underwriter makes a judgmental decision that has the effect of discriminating against a protected class, the outcome should be detected via HMDA testing and remediated. Also, under FCRA and ECOA, a lender is required to give a declined loan applicant an explanation as to why a loan was declined. (aka, the adverse action notice). As such, it is difficult to use opaque, causally challenged unsupervised Machine Learning techniques because of the difficulty in providing a decline reason. Highly transparent traditional models, like FICO score, are often used because of decline reason explainability needs. Also, Credit Machine Learning techniques sometimes use Shapley Values as an approach for explainability.
Fair Lending law implementation could be an example for technology platform companies. The approach could be helpful to drive algorithm transparency via content disclosures and limit unwanted algorithm-induced outcomes (like extreme content or bias) via outcome-based monitoring and correction. The idea is that high tech companies would be responsible for providing quarterly data sets with data specific to unwanted outcomes. An independent party would be responsible for testing the data for evidence of unwanted outcomes. If unwanted outcomes are detected, the offending company would be responsible for remediation and potential redress.
Can technology platform firms police themselves?
Probably not too surprising, the big technology industry's ability to police itself is in doubt. In 2020, a Google AI Ethics and Fairness researcher and leader named Timnit Gebru, was forced out of Google (8). This is an example of the challenge of self-policing and the messy dynamics of profit motives, entrenched culture, industry competition, and the need to implement challenging and sometimes amorphous fairness rules. Frankly, it is hard to fathom that real fairness could be achieved via a self-policing method. Society’s inability to self-police is the underlying premise for government itself. If competitive organizations could self-govern, society would not need governments. As such, we need government to provide laws and regulations to create fairness guardrails and to enforce a level market playing field.
"There was this hope that some level of self-regulation could have happened at these tech companies. Everyone's now aware that the true accountability needs to come from the outside - if you're on the inside, there's a limit to how much you can protect people!"
Deborah Raji, fellow at the Mozilla Foundation
Conclusion
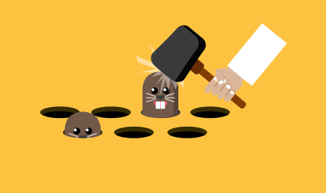
Clearly, technology platform firms, and society at large, need to better manage technology platform content. Industry suggested content moderation strategies may be inadequate. These content moderation strategies, applied outside the realm of machine learning algorithms, are like playing a never-ending and never winning game of whack-a-mole. A more practical approach is to regulate, via a consistent and level market playing field, the platform engagement driving algorithms. We presented two operating example approaches. As these and other approaches are considered, an important consideration is maintaining the freedom of expression. The controlling of the algorithms must maintain our freedoms while limiting the hacking of the human mind as it relates to driving extremism.
While we need to be careful about “not throwing the baby out with the bath water,” being mindful of technology platform firms’ algorithm objectives is also necessary. It is certainly possible to augment the algorithm predictor to a more society friendly objective, like information value as presented in the speed bump example. As Kathy O’Neil, the author of Weapons of Math Destruction, put it:
“With political messaging, as with most WMDs [Weapons for Math Destruction], the heart of the problem is almost always the objective. Change that objective from leeching off people to helping them, and a WMD is disarmed -- and can even become a force for good.”
Notes
(1) Rathje, Van Bavel, van der Linden, Out-group animosity drives engagement on social media, 2021
(2) For a primer on the political-based implications of digital advertising channels, please see our article How Would You Short The Internet?, 2021
(3) Hulett, Our Brain Model, 2021
(4) Thematically, Ellen Ullman addresses algorithm bias in her book, Life In Code, 2017. As an example, she writes: “Far from muting the social context, the internet is an amplifier. It broadcasts its value-laden messages around the world.”
(5) Lewis, Flash Boys, 2014
(7) See the Consumer Financial Protection Bureau for a detailed description of related consumer and mortgage regulation. The following are common pronunciations:
HMDA (commonly pronounced “Hum—dah”)
FCRA (alternatively pronounced “Fic—rah”)
ECOA (alternatively pronounced “Ee - coh - ah”)
(8) Dimosite, The Exile, 2021, Wired Magazine.
Related articles:
Hulett, Preventing the 2021 U.S. Capitol attack, 2021, The Curiosity Vine
Comments